Optimasi Akurasi Model Decision Tree Menggunakan Random Forest Regression untuk Prediksi Kuantitas Pembelian Barang pada Perusahaan Manufaktur
Abstract
Decision Tree merupakan algoritma pengklasifikasian yang sudah teruji untuk pengambilan keputusan dalam banyak bidang permasalahan.Permasalahan pembelian dan jumlah kapasitas gudang di sektor manufaktur sangat riskan. Akurasi jumlah pembelian harus dikontrol sehingga dapat mengurangi resiko over kapasitas gudang. Untuk mendapatkan jumlah pembelian dan kapasitas yang ideal perlu identifikasi dan klasifikasi secara detail faktor-faktor permasalahan sistem pembelian dan pengelolaan gudang. Dari hasil penelitian model Decision Tree mampu memprediksi jumlah quantity pembelian akan tetapi pada data yang tidak berpola tingkat akurasi sangat kurang baik. Berbeda dengan metode Random Forest regression model ini mampu menunjukkan performa dan akurasi yang baik pada data yang berpola ataupun data dengan pola acak. Optimasi dengan importance variabel mampu meningkatkan akurasi menjadi 94,87% dari model random forest regression.
Keywords
Teks Lengkap:
PDFReferensi
[1] Rokach L, Maimon O. Decision Trees. Lecture Notes in Mathematics. 2008;1928:67–86. Lolli F, Ishizaka A, Gamberini R, Balugani E, Rimini B. Decision Trees for Supervised Multi-criteria Inventory Classification. Procedia Manuf [Internet]. 2017;11(June):1871–81. Available from: http://dx.doi.org/10.1016/j.promfg.2017.07.326
[2] Gangsarwijaya N, Anindhita R, Widaningrum DL. Decision tree analysis approach to determine factors that affect the quote order lead time fulfillment. IOP Conf Ser Earth Environ Sci. 2020;426(1). Biau G, Scornet E. A random forest guided tour. Test. 2016;25(2):197–227.
[3] Farnaaz N, Jabbar MA. Random Forest Modeling for Network Intrusion Detection System. Procedia Comput Sci [Internet]. 2016;89:213–7. Available from: http://dx.doi.org/10.1016/j.procs.2016.06.047
[4] Kirasich K;, Smith T;, Sadler B. Random Forest vs Logistic Regression: Binary Classification for Heterogeneous Datasets. SMU Data Science Review [Internet]. 2018;1(3):9. Available from: https://scholar.smu.edu/datasciencereviewAvailableat:https://scholar.smu.edu/datasciencereview/vol1/iss3/9http://digitalrepository.smu.edu.
[5] Richards, Gwynne. Warehouse Management i. 2011. Sylvester EVA, Bentzen P, Bradbury IR, Clément M, Pearce J, Horne J, et al. Applications of random forest feature selection for fine-scale genetic population assignment. Evol Appl. 2018;11(2):153–65.
[6] Wirth R, Hipp J. CRISP-DM: towards a standard process model for data mining. Proceedings of the Fourth International Conference on the Practical Application of Knowledge Discovery and Data Mining, 29-39.
[7] Proceedings of the Fourth International Conference on the Practical Application of Knowledge Discovery and Data Mining [Internet]. 2000;(24959):29–39. Available from: https://www.researchgate.net/publication/239585378_CRISP-DM_Towards_a_standard_process_model_for_data_mining
DOI: https://doi.org/10.24176/simet.v14i2.9494
Article Metrics


Refbacks
- Saat ini tidak ada refbacks.
Indexed by:


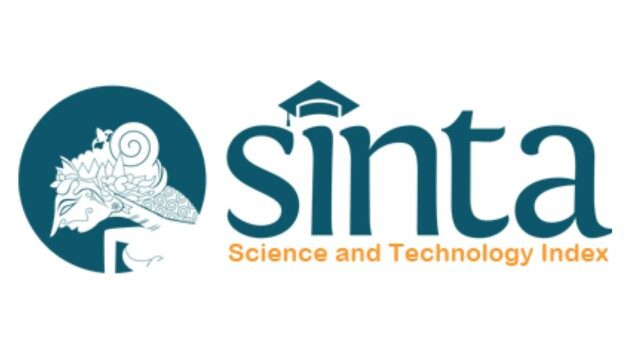
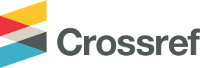
Simetris : Jurnal Teknik Mesin, Elektro dan Ilmu Komputer is licensed under a Creative Commons Attribution 4.0 International License.
Dedicated to:
